Modular AI Chains: How AI and Blockchains Are Merging in a New Web3 Tech Layer
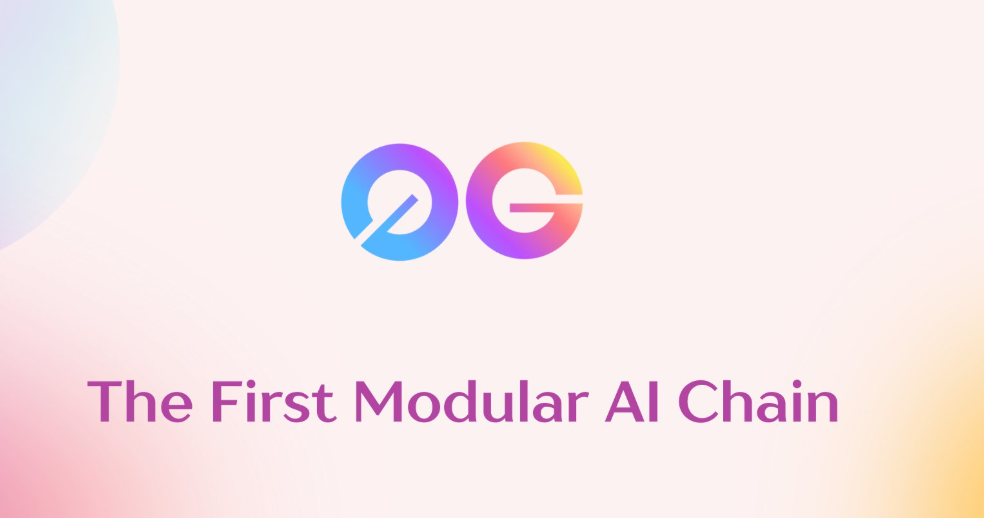
In 2025, the fusion of artificial intelligence and blockchain has gone from a concept to a practice. While previously AI integrations in Web3 were limited to the frontend or experiments with generative art, today we are talking about fundamental changes in the architecture of networks.
Modular AI Chains are entering the scene — blockchains in which AI is built in not as an option, but as a key module.
These are new-generation AppChain platforms where AI models process transactions, make decisions in DAOs, make forecasts, and work with data — all natively, securely, and verifiably.
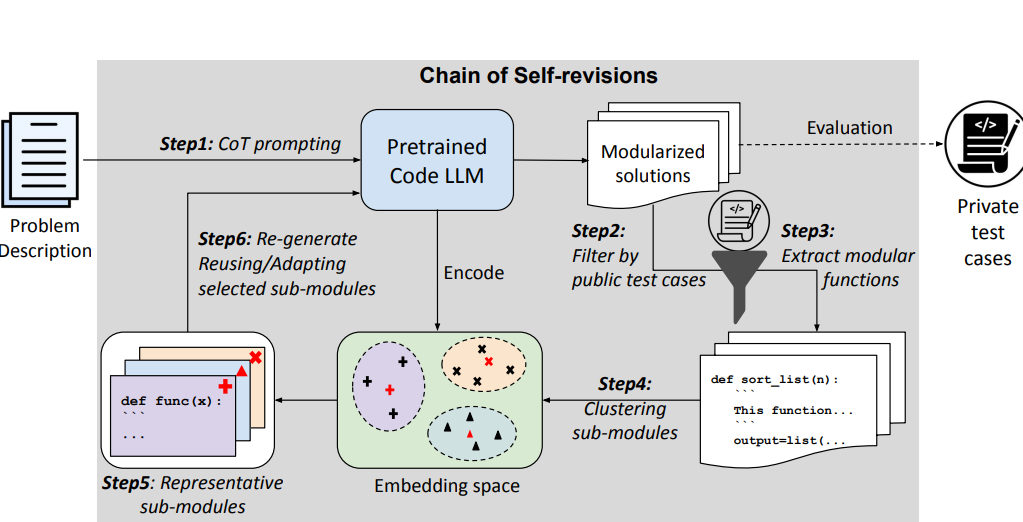
What is Modular AI Chain
Modular AI Chain is not just a network with support for AI tools. This is an infrastructure in which neural networks (usually LLM and ML models) function as full-fledged network participants. Such networks consist of several tiers: contract execution, a separate AI module (runtime), a verification layer, access to data storage, and finalization of calculations.
In this model, developers can directly call AI through smart contracts, verify its output through zk-proofs or TEE, and build an economy on top of it.
Why is this important right now
AI goes beyond API
Today, any Web3 project can connect to GPT or Claude. But these are centralized models. In Modular AI Chains, inference is decentralized, and in some cases, so is training.
Trust in the output appears
The problem of "why did this model give this exact answer?" is solved through provable calculations. zkML allows you to verify that the model really went through the right layers and was not tampered with along the way.
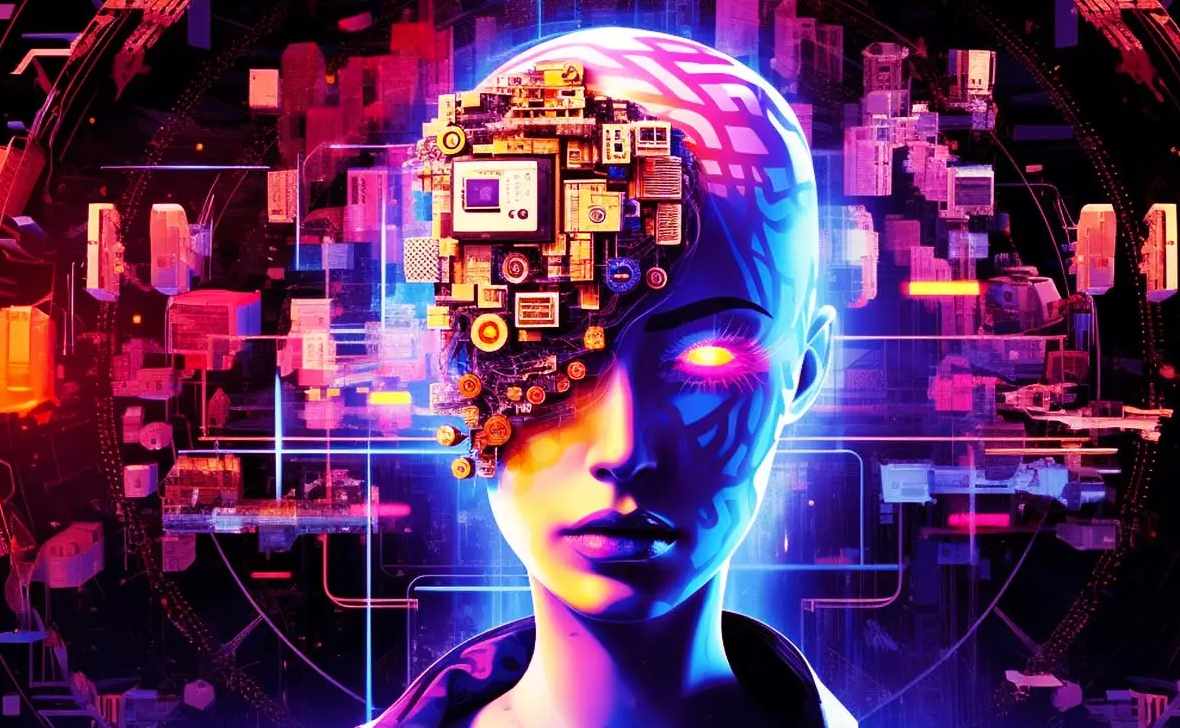
Models and data are assets
In new networks, training data, models themselves, and computing power are tokenized. You can trade access to a model, rent out GPUs, or receive a reward for high-quality datasets.
Who's in the game
Dozens of projects are appearing on the market, each working with different aspects of this idea.
Some focus on zk integration (Modulus Labs), others are creating full-fledged AI infrastructures with tokens (Bittensor), and some are building a DA layer for AI load (0G). There are projects that allow you to run LLM directly inside smart contracts (Giza), and there are those who deal with the privacy of AI queries (Mind Network).
Each of them is taking a step towards a future where neural networks work inside the blockchain as naturally as validators used to work.
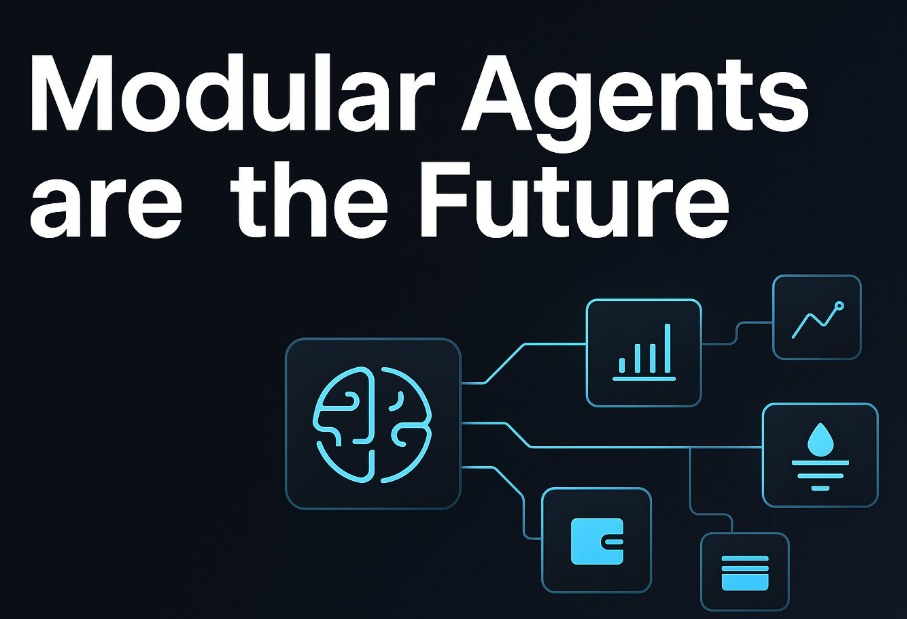
Markets and scenarios
Modular AI Chains allow building:
• decentralized AI assistants built into DAOs or dApps;
• self-governing organizations where AI forms the agenda, proposals, and even voting strategy;
• systems for spam protection, content moderation, and analytics right at the smart contract level;
• DePIN services where AI processes signals from physical devices and responds in real time;
• private requests to neural networks with user data protection.
Key Elements and Features of Modular AI Chains
Element |
Description |
Importance |
Example Projects |
AI
Runtime |
Dedicated
layer for AI inference (e.g., LLMs, ML models) running directly within the
blockchain. |
Facilitates
seamless AI interaction with smart contracts. |
Giza,
Cortex |
zk-Verification |
Zero-knowledge
proofs for verifying AI outputs and ensuring trustworthiness. |
Ensures
the integrity of AI outputs and prevents manipulation. |
Modulus
Labs, Fairblock |
Data
Availability Layer |
A layer
for storing and accessing decentralized datasets crucial for AI training and
inference. |
Ensures
AI models can access vast, decentralized datasets. |
Celestia,
EigenDA, 0G |
Decentralized
Compute |
Distributed
computing power for training and running AI models. |
Reduces
reliance on centralized cloud providers. |
Bittensor,
0G, Mind Network |
Turing-Complete
Contracts |
Smart
contracts that can directly interact with AI models (querying and processing
results). |
Enables
the execution of complex logic based on AI output. |
Giza,
Modulus Labs |
Privacy
Layer |
Protection
of sensitive data during AI computations (using TEEs or zk). |
Prevents
leakage of private information during AI processing. |
Mind
Network, Fairblock |
Tokenization |
Tokens
representing access to models, data, or computing power. |
Creates
an economy around AI models and decentralized datasets. |
Bittensor,
Cortex, Modulus Labs |
AI-Driven
DAO Governance |
Decentralized
autonomous organizations using AI for decision-making and voting. |
Enhances
the efficiency and autonomy of governance systems. |
Cortex,
Modulus Labs |
Inference
Costing |
Pricing
models based on the resources required for AI inference (compute and data). |
Introduces
a sustainable business model for AI use in Web3. |
Bittensor,
Giza |
What challenges lie ahead
Despite the enthusiasm, the industry faces serious challenges.
Firstly, zkML is still expensive and slow. Checking even a small model can take minutes and cost tens of dollars. The infrastructure is still learning to optimize such operations.
Secondly, the privacy problem has not been solved. LLMs tend to remember user input, which means we need mechanisms to ensure that sensitive information does not leak out.
Third, the GPU and inference infrastructure is still concentrated in the hands of centralized providers. The transition to decentralized computing will take time, standards, and mechanisms to protect against bad actors.
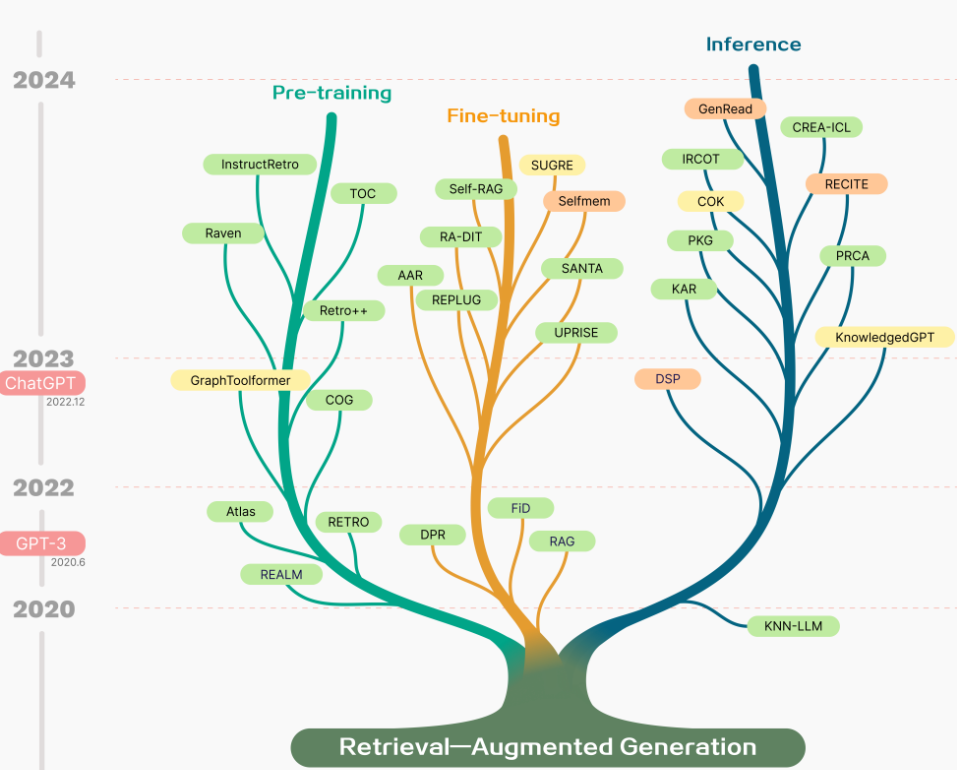
Conclusion
Modular AI Chains are not just a new type of blockchain. They are a fundamental reboot of the idea of what a smart contract can do.
AI is no longer an external assistant, but an embedded element of trusted computing. We are moving towards an architecture where the blockchain does not simply record facts, but can interpret, predict, and act on data in real time.
For developers, this is a chance to build more powerful and autonomous applications.
For investors, this is a marketplace with a new form of tokenized value: models, data, computation.
And for the industry as a whole, this is the path to Web3, which not only stores data, but also mentally works with it.
Comments ()