Privacy-Enhancing Technologies: Safeguarding Data in a Connected World
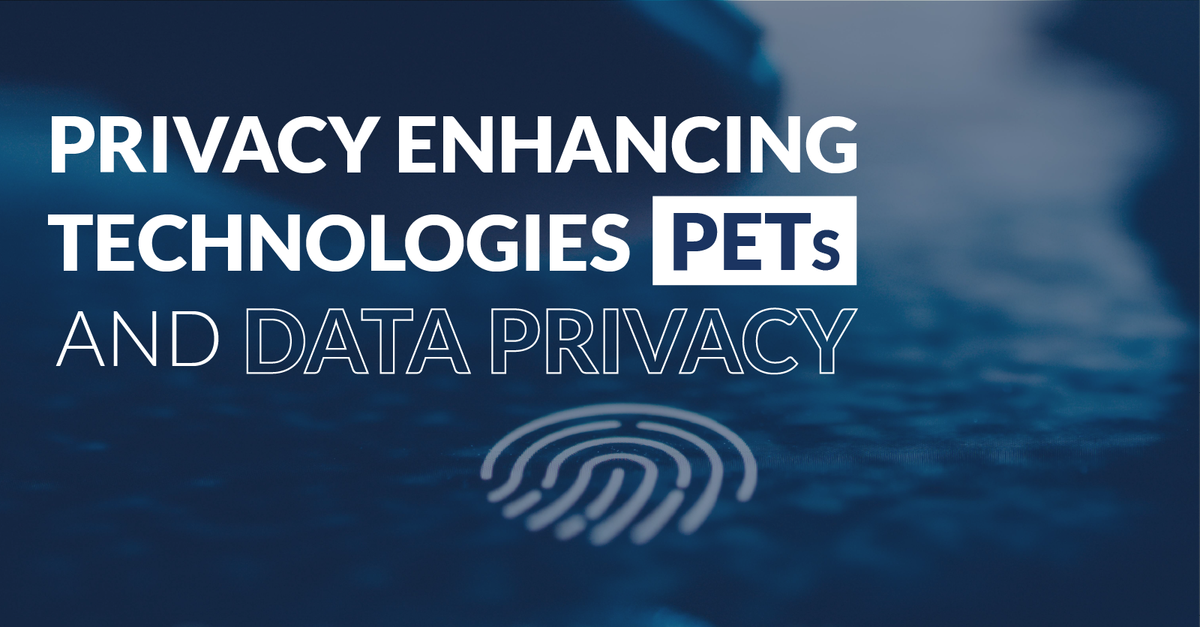
Introduction
In an era where data is the core of innovation, protecting personal privacy has become a critical challenge. Privacy-Enhancing Technologies (PETs) offer a powerful solution, enabling secure data use while minimizing risks to individual privacy. From healthcare to cryptocurrency, PETs are transforming how businesses and individuals handle sensitive information. PETs aren't just tools for compliance, they are catalysts for trust and innovation in a data-driven world.
What are Privacy Enhancing Technologies
Privacy-Enhancing Technologies (PETs) are a series of tools and protocols designed to protect personal data during its collection, storage, processing, and transmission. By minimizing data use, maximizing security, and empowering individuals to control their personally identifiable information (PII), with the ability to reduce the amount of personal data held, ensuring compliance with stringent regulations. In crypto, PETs take on a specialized role, obscuring transaction details on inherently transparent blockchain networks. Technologies like zero-knowledge proofs (ZKPs) and ring signatures enable users to transact privately, shielding sender identities, receiver details, and transaction amounts from public scrutiny. Even in traditional data systems or decentralized finance (DeFi), PETs are defined by their commitment to privacy without sacrificing functionality.
Why PETs Matter
The importance of PETs cannot be overemphasized in a world where data breaches cost businesses an average of $4.45 million per incident, and 94% of consumers demand robust data protection. PETs serve three critical functions: Regulatory Compliance: GDPR fines, which exceeded $1.2 billion between 2021 and 2022, underscore the financial stakes of non-compliance. PETs help organizations meet legal requirements by limiting data exposure and ensuring user consent. Secure Data Sharing: By enabling collaboration without compromising sensitive information, PETs foster partnerships often dubbed “Trust Technologies.” This is vital in sectors like healthcare, where hospitals generate 50 petabytes of data annually, 97% of which remains unused due to privacy concerns. Preventing Breaches: High-profile incidents demonstrate the reputational and financial risks of data exposure. PETs reduce these risks by minimizing stored data and enhancing security. In cryptocurrency, PETs are equally crucial. Blockchain’s public ledger, while transparent, can expose sensitive financial details, making privacy tools like ZKPs essential for protecting users from surveillance, identity theft, and targeted attacks. Moreover, PETs enhance the fungibility of digital assets, ensuring all units of a currency remain indistinguishable and valuable—a cornerstone of their utility as a medium of exchange.
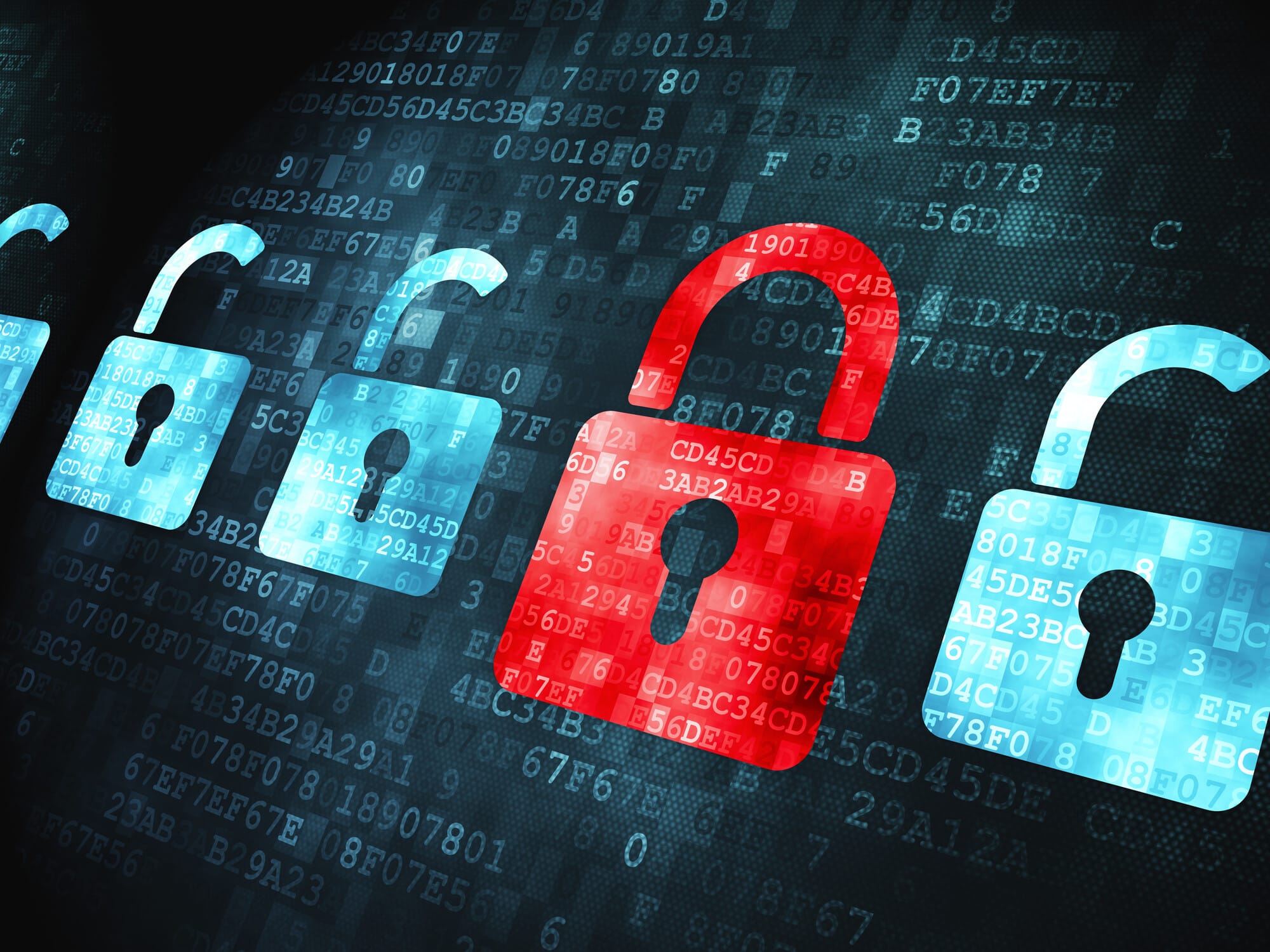
Categories of PETs
PETs can be broadly categorized based on their approach to trust and data minimization:
Soft PETs: These assume a level of trust in third parties and focus on compliance and auditing. Examples include:
- Access Control: Restricting data access to authorized users.
- Differential Privacy: Adding noise to datasets to protect individual identities while enabling group-level analysis.
- Tunnel Encryption (e.g., SSL/TLS): Securing data during transmission.
Hard PETs: These minimize data collection and eliminate reliance on trusted parties. Examples include: - Onion Routing (e.g., Tor): Anonymizes internet traffic through layered encryption. - Virtual Private Networks (VPNs): Masking IP addresses for secure browsing. - Secret Ballot Systems: Ensuring voter privacy in elections. In cryptocurrency, hard PETs dominate, with technologies like ZKPs and ring signatures prioritizing trustless privacy to align with the decentralized ethos of blockchain.
Types of PETs and Their Applications
PETs encompass a diverse range of technologies, each tailored to specific privacy needs. • Differential Privacy: Adds noise to datasets to protect individual identities while enabling aggregate analysis. Used by tech companies to analyze user behavior and in healthcare for secure clinical research. • Homomorphic Encryption: Allows computations on encrypted data without decryption, which is ideal for secure cloud-based analysis in finance and healthcare. • Secure Multiparty Computation (SMPC): Distributes computation across systems to limit data visibility, enabling private data science and DeFi collaborations. • Zero-Knowledge Proofs (ZKPs): Validates information without revealing underlying data. In crypto, zk-SNARKs power Zcash, ensuring transaction privacy, while zk-Rollups enhance Ethereum’s scalability and confidentiality. • Federated Learning: Enables collaborative AI model training without centralizing data. Google’s Gboard uses it to improve autocorrect, and crypto platforms apply it for decentralized analytics. • Ring Signatures: Obscures the sender in a group of potential signers, as seen in Monero’s default transaction privacy. • Synthetic Data Generation: Creates artificial datasets mimicking real data for testing and research, widely used in healthcare and crypto to simulate transactions without exposing real user data.
Type |
Description |
Use Case |
Differential Privacy |
Adds noise to datasets to identify group patterns
while maintaining anonymity. |
Tech companies analyze user data for insights
without compromising privacy. |
Homomorphic Encryption |
Enables computations on encrypted data, results
remain encrypted until decrypted. |
Cloud providers analyze encrypted data without
seeing plaintext, ensuring privacy. |
Secure Multiparty Computation (SMPC) |
Distributes computation across systems, limiting
data visibility. |
Private data science, federated learning, enabling
collaboration without data sharing. |
Zero-Knowledge Proofs (ZKPs) |
Validates information without revealing proving
data, used in identity auth. |
Authenticate age without disclosing date of birth,
used in digital identities. |
Federated Learning |
Devices collaboratively learn a shared model, data
stays local. |
Google uses in Gboard on Android to improve
autocorrect without data collection. |
Generative Adversarial Networks (GANs) |
Generates synthetic data mimicking real data sets. |
Find anomalies in medical tests, network traffic,
using artificial data for research. |
Pseudonymization/Obfuscation/Data Masking |
Replaces sensitive data with fictitious data. |
Protect users' sensitive data, comply with privacy
laws in test environments. |
On-device Learning |
Analyzes user actions on-device, no data sent to
remote server. |
Apple's Face ID uses for accurate, secure face
identification, keeping data local. |
Synthetic Data Generation |
Creates artificial data with same statistical
characteristics as real data. |
Test environments, AI/ML, reduce data sharing,
especially in healthcare research. |
Each type addresses specific privacy needs, from anonymizing data for analysis to enabling secure computations on encrypted datasets. For instance, homomorphic encryption is computationally intensive but allows for secure cloud-based analysis, while federated learning reduces centralized data storage, enhancing privacy in AI training.
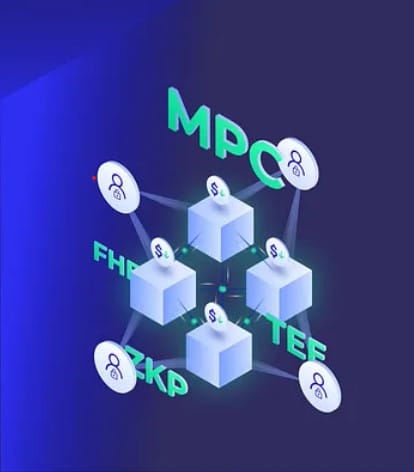
Applications of PETs
PETs are applied across various sectors to enhance privacy while enabling data use. These applications demonstrate PETs' versatility in addressing privacy challenges across industries, from financial transactions to healthcare services. • Data Sharing and Collaboration • Machine Learning and AI • Advertising and Marketing • Healthcare
Challenges and Considerations
Implementing PETs comes with its own set of challenges, which highlights the need for ongoing research and development to make PETs more accessible and effective. • Balancing Privacy and Utility • Regulatory Compliance • Implementation Complexity
Conclusion
Privacy Enhancing Technologies are vital in today's data-driven world, offering a way to protect personal privacy while leveraging the power of data. From differential privacy to federated learning, PETs provide a range of tools to safeguard data across various applications, addressing challenges like balancing privacy with utility and ensuring regulatory compliance. As technology advances, PETs will continue to evolve, shaping how data is collected, used, and protected in an increasingly connected world.
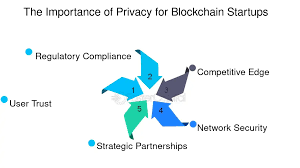
About Mitosis
X
Discord
Mitosis App
Mitosis Docs
Comments ()