The Intersection of Web3 and Artificial Intelligence: Opportunities and Challenges
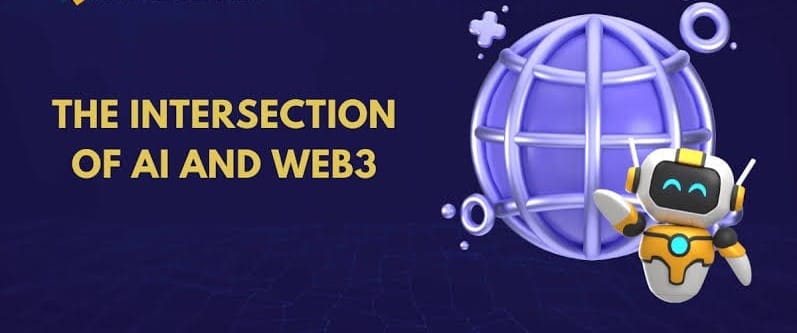
Introduction
We’re standing at the brink of a technological inflection point.
Web3 the decentralized, blockchain-powered vision of the internet is rapidly evolving. Simultaneously, Artificial Intelligence (AI) is undergoing a renaissance, with models like GPT, autonomous agents, and advanced machine learning systems reshaping everything from art to economics.
Individually, these two technologies are potent. Together, they promise to reshape digital infrastructure in ways we’ve only begun to imagine.
At first glance, Web3 and AI might appear to be strange bedfellows. One thrives on decentralization, transparency, and community governance; the other on complex algorithms, centralized compute power, and massive data sets. But their convergence is no longer theoretical, it’s happening now, and it’s producing profound implications for how we build, interact with, and govern the next generation of digital systems.
This article explores the fertile ground where Web3 and AI intersect. We’ll examine their philosophical synergy, emerging use cases, infrastructure projects bridging both worlds, and the serious challenges that must be addressed. Most importantly, we’ll look at what this means for builders, users, and the future of the internet itself.
The Philosophical Alignment: Decentralization Meets Intelligence
At their core, both Web3 and Artificial Intelligence are revolutions not just in technology, but in how we understand agency, trust, and decision-making in the digital world.
Web3: Redefining Trust with Code
Web3 was born from a desire to wrest control away from centralized authorities tech monopolies, financial institutions, and opaque algorithms and return it to users. It replaces intermediaries with smart contracts, relies on consensus instead of trust, and promotes open ownership and transparency.
It’s not just about crypto. It’s about new forms of social coordination, value creation, and self-sovereignty.
AI: From Data to Autonomous Agents
AI, meanwhile, is about making machines that can learn, adapt, and make decisions. It's less concerned with decentralization and more with optimizing intelligence often trained on centralized data and controlled by a handful of corporations.
However, as AI evolves, so does the conversation around democratizing access to AI models, ensuring fairness in algorithmic decision-making, and governing autonomous systems. These are inherently Web3 problems.
Why They Need Each Other
At a deeper level, the ethos of Web3 open-source, censorship-resistant, user-owned aligns with a growing desire to make AI less extractive and more accountable. Without such a shift, AI risks becoming a black box serving elite interests. Web3 can serve as the checks and balances system AI desperately needs.
At the same time, AI can make Web3 smarter. It can help DAOs govern more efficiently, enhance DeFi risk modeling, or improve fraud detection in real-time. It brings intelligence to the rigid logic of smart contracts.
In short: Web3 gives AI structure, values, and distribution. AI gives Web3 adaptability, learning, and autonomy.
Real-World Use Cases at the Intersection of Web3 and AI
While the fusion of Web3 and AI is still in its infancy, several promising use cases are already taking shape some speculative, others live in production. These use cases fall into several broad categories that reflect the unique strengths of each domain.
1. Decentralized AI Marketplaces
Imagine a world where anyone can contribute to and access AI models without going through Big Tech gatekeepers. Platforms like Ocean Protocol and Fetch.ai are leading this vision by enabling:
- Data sharing without ownership compromise: AI models can be trained on decentralized datasets where data remains private and is monetized securely.
- AI-as-a-service on-chain: Developers can publish models as decentralized services callable through smart contracts and paid for in crypto.
- Incentivized collaboration: Contributors can be rewarded for improving models or providing training data, creating a decentralized R&D pipeline.
2. Autonomous Agents for DeFi and DAOs
AI-powered autonomous agents are already changing how decentralized organizations and finance systems operate. These agents can:
- Optimize liquidity and yield farming strategies in real-time by learning market conditions.
- Auto-balance DAO treasuries based on predefined risk tolerances.
- Make governance smarter by analyzing proposals, predicting outcomes, and surfacing risks before votes go on-chain.
Projects like Autonolas and Numerai blend collective intelligence with blockchain-backed economic incentives to drive smarter decision-making.
3. Enhanced Identity and Reputation Systems
In a pseudonymous Web3 world, trust is scarce. AI can help fill the gap by:
- Building dynamic reputation scores using on-chain activity, voting behavior, and contribution history.
- Detecting bots and Sybil attacks by analyzing behavioral data and social graph anomalies.
- Enabling verified credentials through zero-knowledge proofs and AI-backed attestations without revealing sensitive data.
This kind of synergy could radically improve Web3 UX and trust layers without compromising privacy.
4. Fraud Detection and Smart Contract Security
Security remains one of the biggest challenges in crypto. AI has the potential to:
- Analyze contract patterns to detect exploits before they’re executed.
- Flag abnormal transactions and wallet behavior in real time.
- Assist auditors by scanning contracts for vulnerabilities with natural language and code understanding.
Several emerging platforms are integrating AI into security layers, creating a more proactive defense system for the ecosystem.
5. Creative Collaboration and Content Generation
Web3 has already birthed a new creative economy through NFTs and decentralized publishing. AI enhances this by:
- Enabling generative art and music tailored to buyer preferences.
- Assisting writers and designers in creating Web3-native media and interfaces.
- Creating adaptive in-game economies and NPCs for decentralized gaming.
Some NFT projects even use AI to evolve avatars or personalize them based on user engagement or ownership history.
The Challenges of Convergence
As promising as the marriage of Web3 and artificial intelligence may seem, it’s far from frictionless. The integration of these two transformative technologies exposes critical tensions technological, ethical, and operational that innovators must address before the fusion can scale.
1. Data Privacy vs. Data Availability
AI thrives on data. Web3 champions privacy and decentralization. This creates an inherent paradox:
- Training AI models requires large, labeled datasets — yet in Web3, data is either permissionless (on-chain) or encrypted and siloed (off-chain).
- User data ownership in Web3 means individuals may not consent to contribute their data for AI use, complicating model training.
- Solutions like federated learning and zero-knowledge machine learning (zkML) offer promise but remain nascent and technically complex.
2. Computational Constraints of Blockchain
Blockchain systems, especially Layer 1s, are inherently resource-limited due to decentralization:
- Running large AI models on-chain is virtually impossible with today’s infrastructure.
- Even running lightweight inference tasks can strain networks or bloat gas fees.
- Most AI computations must still be done off-chain, raising concerns about verifiability and trustlessness.
Emerging platforms like Cartesi, Aleph.im, and Modulus Labs are experimenting with bridging this gap, but it’s still early.
3. Governance and Control
Who decides how AI models are trained, updated, and deployed in a decentralized ecosystem?
- AI systems are complex and often opaque creating a “black box” problem. When these models drive decision-making in DAOs or DeFi, accountability becomes murky.
- Decentralized governance isn’t well-equipped to manage fast-evolving AI models. Updating models requires expertise, rapid iteration, and sometimes centralized coordination all antithetical to the DAO ethos.
Balancing openness with efficiency is a governance challenge yet to be solved.
4. Ethical Dilemmas and Bias
Bias in AI is already a critical issue but decentralization doesn’t eliminate it. In fact, it may complicate it:
- Without clear oversight, AI models deployed in decentralized contexts might reinforce systemic biases or amplify misinformation.
- Decentralized models of content moderation or decision-making are prone to mob rule or manipulation, especially when AI is involved.
- Web3’s anonymity makes accountability harder, exacerbating risks when AI is weaponized or behaves unpredictably.
Responsible AI frameworks need to be adapted to decentralized contexts something no one has yet cracked.
5. Security and Exploit Risk
- AI systems interacting with smart contracts introduce new attack surfaces like manipulated training data or adversarial prompts.
- On-chain AI agents could be tricked by malicious actors, leading to unintended transactions or governance votes.
- Inversely, AI models used for security (e.g., fraud detection) can be reverse-engineered and bypassed if not properly secured.
The stakes are high: a compromised AI could mean lost funds, corrupted governance, or systemic vulnerabilities.
Key Projects and Innovators Bridging the Gap
The convergence of Web3 and AI has spurred a new wave of experimentation and innovation. From decentralized AI marketplaces to on-chain inference engines, the frontier is rich with visionaries pushing boundaries. Below are some of the standout players and initiatives shaping the space:
1. Ocean Protocol – Decentralized Data Sharing for AI
Ocean Protocol enables data owners to share and monetize data securely and privately using blockchain. For AI developers, this is a game changer:
- Tokenized data assets allow creators to publish and sell datasets as NFTs or ERC20 tokens.
- Developers can access previously siloed datasets to train machine learning models.
- Ocean’s focus on data sovereignty aligns perfectly with Web3’s privacy-first values.
This kind of infrastructure could fuel a marketplace for high-quality AI training data that respects user consent and ownership.
2. Fetch.ai – Autonomous Agents on a Decentralized Network
Fetch.ai builds autonomous AI agents that operate in decentralized environments:
- These agents can negotiate, transact, and collaborate on behalf of users.
- Use cases span decentralized logistics, smart cities, and DeFi optimization.
- The protocol is underpinned by a bespoke blockchain tailored for high-throughput AI operations.
Think of Fetch as creating a “digital workforce” of intelligent bots that act within a Web3 logic system.
3. Bittensor (TAO) – Incentivizing Open AI Models
Bittensor is one of the most ambitious projects merging AI and crypto:
- It’s a decentralized machine learning network where participants train AI models and are rewarded based on performance.
- Models contribute to a collective “brain” and are ranked in real-time through a staking mechanism.
- This aligns incentives and allows open-source models to compete and collaborate without centralized control.
Bittensor could evolve into a decentralized alternative to OpenAI, but with a native economy and transparent governance.
4. Gensyn – Decentralized Compute Marketplace for AI
Training AI models requires immense compute power, often monopolized by a few cloud providers. Gensyn disrupts this by offering:
- A decentralized network of idle hardware (GPUs, TPUs) where anyone can contribute and earn.
- Verifiable training jobs executed off-chain, with proofs stored on-chain.
- It reduces AI development costs and aligns with Web3’s democratization ethos.
Imagine training a large model using a decentralized grid of contributors permissionlessly, and without AWS.
5. SingularityNET – A Marketplace for AI Services
Led by renowned AI researcher Dr. Ben Goertzel, SingularityNET aims to be the Amazon of AI services, but decentralized:
- Developers can deploy and monetize AI algorithms via smart contracts.
- Users can combine services into more complex pipelines (e.g., speech-to-text + translation + sentiment analysis).
- AGIX, its native token, governs the ecosystem.
It’s a bold attempt to decentralize access to advanced AI capabilities and empower global developers.
6. Modulus Labs & zkML – On-Chain Verifiable AI
One of the most pressing challenges is how to verify that off-chain AI inference was done correctly. Modulus Labs is tackling this via:
- Zero-knowledge machine learning (zkML): proving an AI model’s output without revealing the inputs or weights.
- This opens the door to trustless AI agents interacting with smart contracts and DAOs.
- zkML could become a key pillar of on-chain AI applications.
Think of it as "proof of intelligence" verifiable, privacy-preserving, and permissionless.
7. Arkifi & Ritual – AI-Powered On-Chain Intelligence
- Arkifi is building an LLM (Large Language Model) specifically trained on on-chain financial data to provide intelligent DeFi analysis.
- Ritual is creating decentralized AI agents that can interpret governance, track protocol metrics, and act autonomously across blockchains.
These tools aim to supercharge DAOs, DeFi, and cross-chain coordination with AI-powered insights.
Use Cases Shaping the Future
The fusion of Web3 and AI is not just theoretical. It is actively reshaping how we think about coordination, intelligence, and ownership in digital systems. Here are some of the most compelling real-world use cases emerging at the intersection of these technologies:
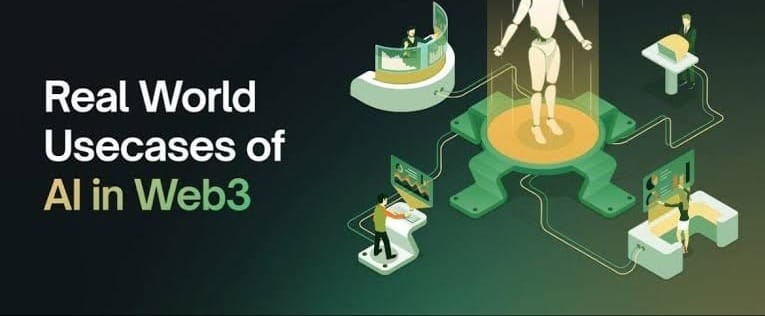
1. Decentralized Autonomous Organizations (DAOs) with AI Agents
AI can make DAOs more efficient and less reliant on human micromanagement by:
- Analyzing governance proposals to surface potential risks or summarize key points.
- Recommending actions based on treasury health or on-chain metrics.
- Automating operations, like yield strategy adjustments or validator selections.
Imagine a DAO where AI helps members make data-driven decisions while still honoring decentralized voting structures.
2. Personalized AI Wallet Assistants
Web3 wallets are becoming smarter. By integrating AI:
- Users can receive investment suggestions based on their portfolio and risk profile.
- Get alerts about airdrops, governance votes, or DeFi liquidation risks.
- Interact with the blockchain through natural language queries, e.g (“Send 0.1 ETH to Alice” or “Swap my USDC for the best yield”).
This evolution turns the wallet from a static interface into an intelligent financial agent.
3. AI-Powered NFT Discovery and Curation
The NFT space suffers from overwhelming noise. AI can help by:
- Recommending NFTs based on user behavior, social sentiment, and market trends.
- Detecting wash trading or suspicious minting activity, protecting collectors from scams.
- Curating dynamic galleries or even generating art in response to community tastes.
Platforms like GigaMart and Zora are experimenting with this, blending machine learning with market signals.
4. AI-Enhanced On-Chain Reputation and Identity
Web3 identity is evolving beyond simple wallet addresses. AI adds nuance by:
- Aggregating a user’s on-chain actions into behavioral profiles.
- Assigning trust scores for lending, DAO participation, or bounty rewards.
- Detecting Sybil attacks or fraudulent bots in community networks.
Tools like Gitcoin Passport and Proof of Humanity could become far more robust with AI analysis layered in.
5. Predictive Analytics for DeFi and On-Chain Markets
DeFi is inherently data-rich and fast-moving. AI brings powerful predictive capabilities:
- Forecasting token price movements using historical data, social media signals, and macro trends.
- Optimizing yield strategies in real-time by adjusting positions based on protocol metrics.
- Stress-testing smart contracts using generative adversarial networks (GANs) to simulate attacks.
These tools enable traders and protocols to act more proactively and reduce risks.
6. Synthetic Data Markets and Model Training
Data is the fuel of AI and in Web3, it can be monetized:
- Projects like Ocean Protocol allow users to sell data or access it for model training.
- Synthetic datasets can be generated using AI, reducing reliance on sensitive or biased real-world data.
- Token incentives can crowdsource labeling, model tuning, and validation.
This could democratize AI development and reduce gatekeeping by big tech.
7. AI + Web3 in Gaming and the Metaverse
The metaverse and gaming sector will likely be one of the biggest beneficiaries of AI-Web3 integration:
- AI can power intelligent NPCs that evolve based on user interactions.
- On-chain assets (NFTs) can be trained, upgraded, or customized by AI behavior models.
- Decentralized AI-generated narratives or art can power dynamic, player-driven storylines.
Games like AI Arena and Altered State Machine are already testing these concepts.
8. AI-Based Security and Threat Detection
Security in Web3 remains a massive challenge. AI can provide:
- Anomaly detection in smart contract behavior, potentially spotting exploits in real time.
- Wallet behavior profiling to detect hacks, rug pulls, or draining schemes.
- Enhanced phishing and scam protection for users.
Companies like Forta Network are pioneering decentralized threat intelligence powered by AI agents.
9. Content Moderation and Governance in Social Protocols
Decentralized social networks like Farcaster or Lens lack traditional content filters. AI can:
- Flag harmful or illegal content without central authorities.
- Rank or surface valuable posts using user-defined moderation preferences.
- Assist with community-based governance, ensuring fair and context-aware enforcement.
AI could enable scalable moderation while preserving the ethos of permissionless platforms.
Ethical and Governance Considerations
While the blend of Web3 and AI promises radical innovation, it also magnifies long-standing concerns about ethics, governance, and power concentration. The decentralized ethos of Web3 can clash or harmonize with the opaque, black-box nature of many AI systems. Navigating these tensions requires deliberate effort.
Transparency vs. Complexity
Smart contracts are public, auditable code. In contrast, many AI models, especially deep neural networks, operate in ways even their creators can't fully explain. Integrating these two introduces the risk of "black-box governance", where decisions in decentralized systems are guided by AI logic that users can’t verify.
To mitigate this, researchers are exploring verifiable machine learning (vML) and zero-knowledge proofs for AI, enabling models to make predictions without revealing sensitive data or inner workings.
Bias and Fairness
AI is only as unbiased as the data it’s trained on. When AI systems interact with Web3 platforms whether moderating content or scoring on-chain reputations biased outputs can propagate across immutable systems, causing long-term harm.
Decentralized oversight, model auditing, and community-driven training datasets may be part of the solution. But we must also acknowledge that biases in AI are social problems first, not just technical ones.
Autonomy and Human Oversight
Autonomous agents in DeFi, DAOs, or marketplaces sound futuristic but who’s accountable when they go rogue? If an AI agent drains liquidity pools due to a faulty strategy or enacts biased moderation, what recourse do users have?
Web3’s permissionless nature adds complexity to this. Future systems may need hybrid frameworks that blend code-level autonomy with community-based vetoes or governance backstops.
AI Centralization in a Decentralized World
One of the most ironic risks: while Web3 aims to distribute power, most AI today is trained and owned by a few centralized entities (e.g., OpenAI, Google, Anthropic). If these models become foundational infrastructure in Web3 applications, we’re rebuilding centralized dependencies within decentralized ecosystems.
Efforts like decentralized AI marketplaces (e.g., Fetch.ai, Bittensor, Gensyn) aim to counterbalance this trend. But they face the dual challenges of scalability and model quality parity with centralized giants.
Conclusion:
The convergence of Web3 and AI signals a new chapter in the evolution of technology one that could redefine how we govern, create, and exchange value.
These technologies offer complementary strengths: Web3 provides verifiable infrastructure, transparent incentives, and decentralized governance. AI brings adaptability, contextual intelligence, and automation. When integrated mindfully, they unlock powerful new use cases: autonomous DAOs, intelligent wallets, dynamic NFTs, decentralized model marketplaces, and more.
But with great power comes great responsibility. This intersection raises urgent questions about transparency, ethics, control, and power. The systems we build today will shape not just digital infrastructure, but the future of collective intelligence and coordination.
If the first wave of Web3 was about decentralizing value, and the first wave of AI was about automating cognition, their union could bring about decentralized intelligence systems that learn, evolve, and act without centralized intermediaries.
The journey is still early, and the path is anything but linear. But one thing is clear: those who understand both paradigms and how to navigate their synergies and trade-offs will be the architects of the next technological frontier.
Comments ()